Artificial intelligence
Customized according to professional fields
Ensemble AI technology
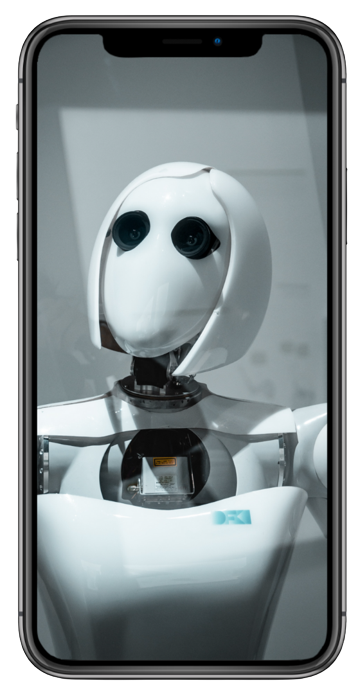
World-class validated
Matchless original technology
AI
20
Prime Quality
Artificial Intelligence
years of
Research & Development
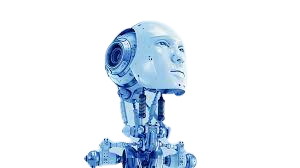
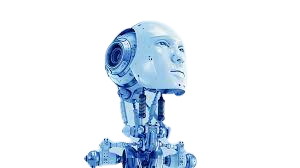
Saltlux Technology’s Artificial Intelligence research focuses on four areas: linguistic intelligence, voice intelligence, learning/reasoning intelligence, and visual intelligence. Meanwhile, the artificial intelligence product that is being commercialized is ensemble AI in which the system is made intelligent through an interaction in which knowledge-based reasoning and data-based are integrated.
In addition, Artificial Intelligence technology needs to be accessible to real-life services. Therefore, the system was trained through many processes, including cognition, understanding, knowledge, reasoning, and prediction with data such as language, voice, and vision. We incorporate the necessary elements into each procedure and improve it to ensure optimum performance. As a result of the convergence of AI technologies, our AI products are maturing into an innovative AI platform that delivers integrated intelligent software solutions and services.
What is outstanding about our Artificial Intelligence TECHNOLOGY?
Deep learning-based intention/meaning understanding that integrates high-precision machine learning-based language cognitive technology and deep learning-based language/intention/knowledge learning. It applies an ensemble deep Q/A technology that combines explicit knowledge expression based on the knowledge base, reasoning, and tacit knowledge learning based on search and deep learning.
Provide world-class comprehension Artificial Intelligence Technology using Saltlux natural language processing, machine learning, and deep learning technologies based on extensive language resources and knowledge graph, a critical resource for semantic analysis.
Provide features that enable humans to create and verify knowledge through a dual-spiral methodology in which machines and humans work together. This allows machines to augment knowledge by collecting and integrating knowledge resources, while also learning new knowledge and making complex inferences.
Provide an environment for interlocking large-scale legacy big data held in the organization, and utilizing data in connection with the company's internal systems, such as KMS and ERP.
Improve quality by building language resources and knowledge data in specific domains, learning or managing models through learning data, and enabling customized service modeling based on client requests and intentions
Main Applications
Our Artificial Intelligence technology can be applied to various fields, applications, and products.
Products/services
AI consulting
Virtual secretarial service
Analysis service
Professional consultation
Intelligent robot
Formats
Big data
Document
Voice
Pictures
Video
Typical Artificial Intelligence Engine
Explore Saltlux Inc.'s signature Artificial Intelligence Engines
Natural Language Understanding Engine LEA
LEA (Language Engineering & Analysis) is a language analysis engine developed based on machine learning/deep learning. It can handle text analysis functions such as morpheme analysis, object name recognition, sentence structure analysis, and emotion analysis for non-structured data processing. Main features:- Natural language processing features
- Intention analysis feature
- Question understanding feature
- Dictionary management feature
- Morpheme analysis feature
- Sentence structure analysis feature
- Object name extraction feature
- Reputation extraction feature
Speech Recognition Engine (Speech-to-text, STT)
The Speech recognition engine provides speech recognition function for various types of services based on speech interfaces. Our Artificial Intelligence speech recognition engine is based on acoustic model adaptive learning sophisticated by Deep Learning and a baseline acoustic model with Long Short-Term Memory (LSTM) technology. The engine is built with multilingual speech recognition and high-quality basic speech recognition models trained with these multilingual speech data. Main features:- Deep neural network-based speech recognition learning
- Large-capacity multilingual speech database learning
- Speech recognition service
- Acoustic model adaptive learning
- Language model learning
- Providing high-quality speech recognition model
Speech Synthesis Engine (text–to–speech, TTS)
Speech synthesis engine learns human voices from given sentences and artificially creates human voices with tone and intonation similar to humans. In particular, speech synthesis can be created with the real-time learned voices of specific individuals or domains using Deep Learning and Tacotron model. Main features:- Learning data management
- Dictionary management
- Learning management
- Service management
AI Chatbot Engine
An intensive dialogue system capable of understanding the exact meaning of the user's speech based on knowledge graphs and complex inference-based natural results. It provides excellent answers, thereby generating a powerful and flexible dialogue model. Main features:- In-depth meaning/intention understanding
- Knowledge graph and reasoning
- Ensemble in-depth Q&A
- Simultaneous multichannel support
- Powerful bot builder and smart card
- Bot group and reutilization of knowledge
Deep Q/A Engine
Deep Q/A engine is a system that finds and presents the best answers to the user's questions from the accumulated knowledge by training it to learn. This is done by integrating knowledge base Q&A (KBQA), information retrieval based Q&A (IRQA), machine answering Q&A (MRCQA), counseling and dialogue history learning-based Q&A (DLQA). Main features:- Knowledge Based Question Answering, KBQA
- Information Retrieval Question Answering, IRQA
- Machine Reading Comprehension Question Answering, MRCQA
- Dialog Learning based Question Answering, DLQA
- Knowledge and language resource building management
- Q&A quality control
- Q&A service management
Machine Translation Engine (MT)
Saltlux's machine translation engine is based on an artificial neural network, which has evolved from Saltlux's existing statistical machine translation engine. It is also integrated and configured with CAT (Computer-assisted translation) tools to specialize in machine translation learning for each domain to improve translation quality and productivity. Main features:- Machine translation learning and model management
- Learning data and dictionary management
- Service management feature
- Translation result confirmation and post-translation editing management
Machine Reading Comprehension Engine (MRC)
The machine reading comprehension (MRC) engine searches relevant documents for an answer to the inquiry. Main features:- Providing optimal machine reading comprehension results by situation
- Can be used as Open QA
- Service expansion in connection with AI engines
- Machine reading comprehension service
- Learning data management
- Learning management
- Model validation and distribution
Complex Reasoning Engine
The complex reasoning engine accumulates knowledge extracted from structured and unstructured documents in the form of knowledge graphs, then generates knowledge by searching and inferring new facts based on given knowledge or relationships between different sets of rules. Especially, it can derive new relationships from existing knowledge and use them as new knowledge by combining logic rule-based deductive reasoning and machine learning-based inductive reasoning. Main features:- Large-scale semantic reasoning
- Heuristic rule-based reasoning
- Spatiotemporal reasoning
- Probability/uncertain reasoning
Knowledge Extraction Engine Kent
Knowledge Extraction from Natural Language Text (KENT) extracts from structured and non-structured data; learns and manages dialogue processing. The automatic knowledge extraction engine can extract useful knowledge information from product manuals, contracts, etc. Main features:- Automatic knowledge extraction task management feature
- Knowledge extraction feature
- Knowledge extraction results supervision feature
- Knowledge learning generation and management feature
- Automatic knowledge extraction model evaluation feature
Image Recognition Engine
The image recognition engine recognizes the image's objects and classifies them based on the recognized result. The image recognition engine supports future applications such as visual-based search, video captioning, autonomous driving, and visual Q&A. Main features:- Semantic Segmentation
- Pose Estimation
- Hand Gesture Recognition
- Face Landmark Detection
- Age-Group/Gender Classification
- Face Recognition and Verification
- Image understanding with knowledge graph
Artificial Intelligence TECHNOLOGY FOR SALTLUX INC's CUSTOMERS
See the engines that apply artificial intelligence technology that Salltux Inc. developed for major customers around the world.
Various customer service channels are expanding in the financial sector, including mobile and AI-based services. As a result, the demand for NH Nonghyup Bank's AI counseling service channels has increased. Their Customer Happiness Center aims to build a system infrastructure that has AI counseling services for counselors and customers, both online and offline, to expand service channels. The Customer Happiness Center is expected to play a pivotal role in extending artificial intelligence services and setting up an example for the entire financial industry.
Project Information
- Customer consultation Q&A service building: provides Q&A service for customer consultation knowledge. The service receives question from the customer via the mobile app chat service, finds the correct answer, delivers it in text form, links to a service such as an account inquiry or transfer.
- Real-time telephone consultation support service building: provides answers to questions by converting counseling content into text in real-time. Unlike other cases in which the counselor asks a question directly, you can quickly respond to customer needs by referring to the AI system's feedback.
- AI Virtual Counseling Service "Callbot" building: The AI system first receives the customer's call and provides a simple answer or connects to a professional counselor. Interactive counseling is provided through a dialogue model while usual questions are answered by the Q&A system.
- Guide Robot Consultation Service: provides customer consultation and guidance services in which a guide robot connects with APIs provided by the Nonghyup Bank AI system. Consultation is offered to customers via setting up a dialogue model with consultation contents and connecting with the Q&A system.
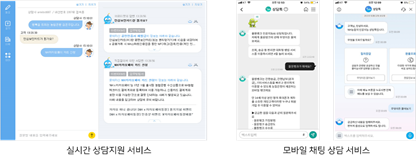
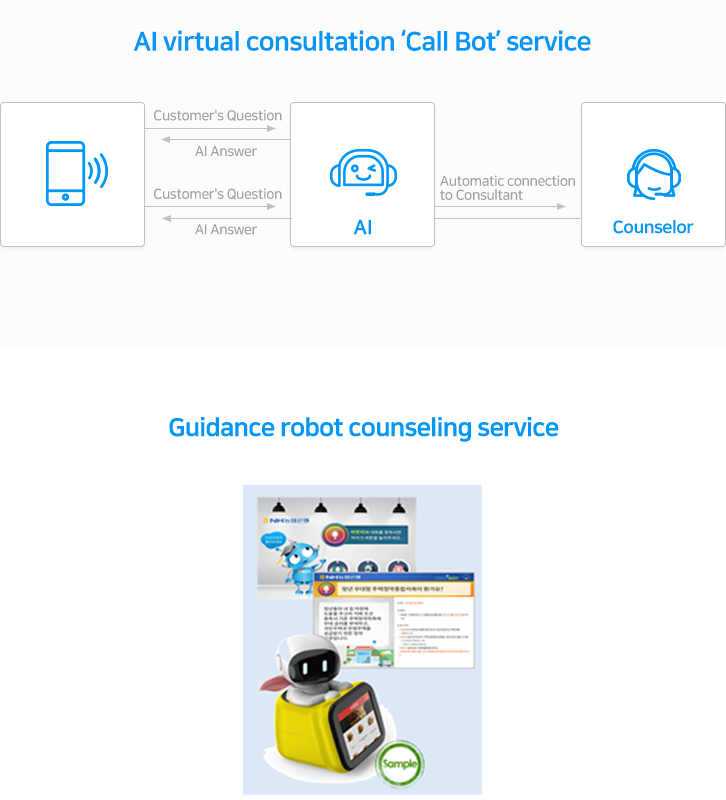
Application technology and solutions
Consultation services adapted AI technologies such as natural language understanding engine, deep QA engine, dialogue processing engine, and machine reading comprehension engine to process conversation flow and provide Q&A for service scenarios.
Main accomplishments
- Building a system infrastructure in which services are expanded across all channels to employees and customers through the AI counseling system.
- Interactive consultation service is provided through deep QA engine and dialogue processing engine. Through dialogue, the system can identify, answer customer intentions, improve the ability to answer simple questions that are not clear, and consult with additional questions to provide smooth services.
- Enhancing counseling quality and specializing counselors' duties by providing counseling assistants.
- Expanding the service to general users by installing the consultation service in the Nonghyup Bank mobile apps, including All-One Bank and Smart Banking App.
- Korea's first AI telephone call service - Call Bot, was developed and commercialized. It can achieve a faster response than the existing ARS guidance while also allowing the counselor to concentrate on professional counseling. The pilot robot system could provide interactive counseling and knowledge services through the operation of the guide robot in offline channels such as branches.
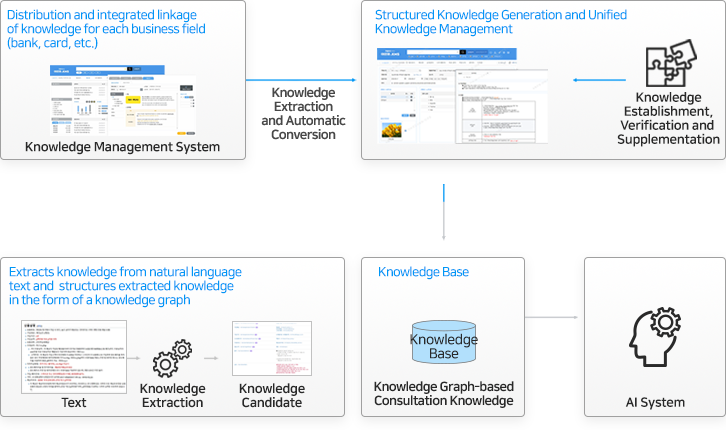
Project Information
- Knowledge Content Creation Management: In consideration of knowledge extraction and conversion into a knowledge graph, work categories are defined in a hierarchical structure. In addition, knowledge contents for each category are managed and created via building a detailed content management tool.
- Automatically Extract Knowledge Data: It automatically extracts knowledge data candidates by analyzing knowledge content written in unstructured text. This is an automatic knowledge extraction process in which the target knowledge is identified through natural language processing, and the data is extracted through deep learning-based learning models. The extracted knowledge data is converted into a triple form to be stored in the knowledge graph.
- Knowledge learning verification and management: Building a quality management environment where the automatically extracted knowledge data can be verified and edited. Constant improvement of quality and performance in automatic knowledge extraction.
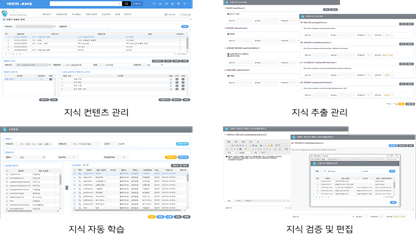
Application technology and solutions
In order to extract and manage knowledge from knowledge content, a natural language understanding engine, knowledge learning engine, and complex inference engine are applied.
Main accomplishments
- Automatic knowledge extraction and knowledge extraction are technical fields that still require a lot of effort to research and develop. Various methods, including language processing and machine learning, were studied to improve the quality and satisfy the results of the necessary knowledge extraction from the domain's contents. It could be regarded as a successful example of applying automatic knowledge extraction to real-life services.
- Knowledge content managed in the knowledge management system can be utilized in the artificial intelligence system, thereby increasing the use of knowledge. In addition, unifying the knowledge information that has been managed separately reduced the cost of knowledge maintenance and made it easier to use.
KEPCO KDN introduced Chatbot services, which applied basic dialogue processing engines such as high-performance natural language processing, intent/entity mapping technology, and dialogue modeling. Efforts have been made to extend the system to provide effective Q & A for a variety of future knowledge, including linking knowledge graphs. The services were based on an easy-to-access messenger platform for the convenience of KEPCO's employees. The goal is to provide personalized answering services beyond simple knowledge in fields as business trips, ICT equipment rentals, and connections to workers who need work contact.
Project Information
- Domain dictionary building: collecting frequently asked questions from employees and building a terminology dictionary.
- Work process dialogue model building: building a dialogue model so that Chatbot can operate according to each work process.
- Sentence analysis: applying and treating various intents and sentences derived from consultations separately to increase the hit rate in intent extraction.
- Guidance service implementation: building business trip expense guidance and ICT equipment rental work guidance.
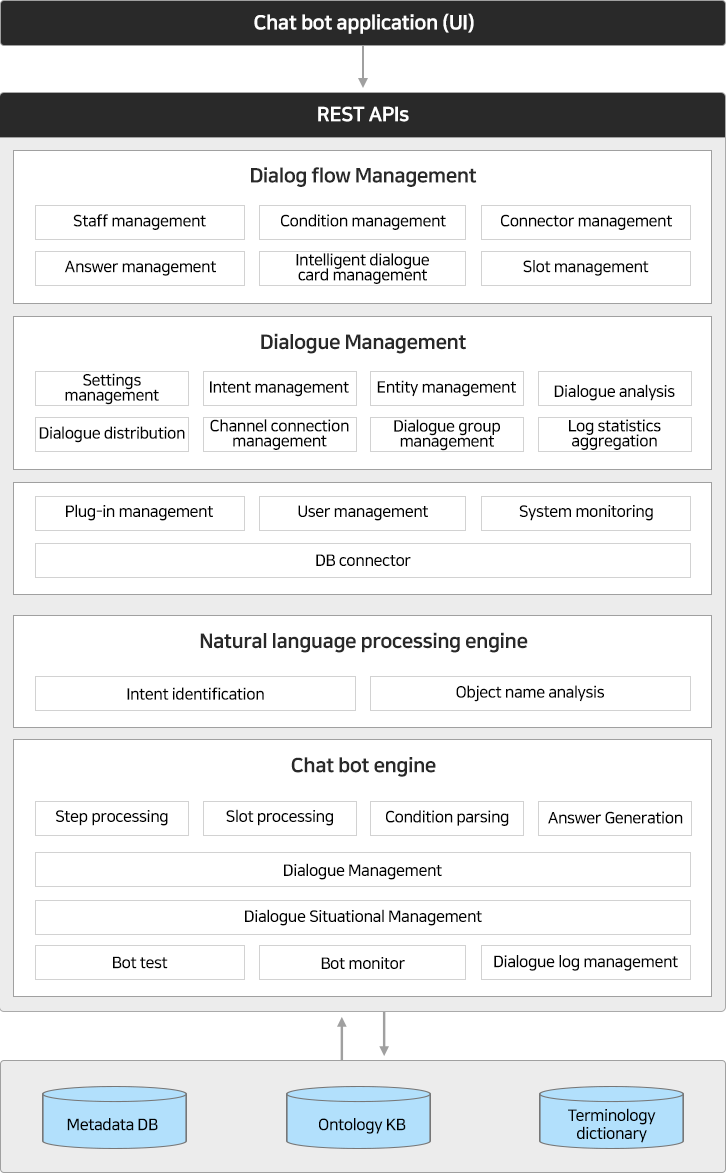
Application technology and solutions
AI Suite’s dialogue processing engine and selective application of deep Q/A technology.
Main accomplishments
- Improving work efficiency by reducing human resources for simple jobs with the help of knowledge-based work support.
- Creating complex logical flow charts to take specific actions for different work situations.
- Providing not only Q&A about business procedures but also business applications and reservations through groupware interworking on one messenger platform.
Due to the open technology environment, service environment such as broadcasting/communication integration, and the evolved content consumption structure, there is an increasing need for an environment where necessary content can be easily found and consumed. That's why we developed a customized content recommendation technology based on the user's characteristics and situations. In this example, we explored and developed a user-tailored broadcast content recommendation search for broadcast video and related content using language analysis, search, mining, and semantic technologies.
The broadcast content recommendation search system is composed of four systems, including data collection, content search, content recommendation, API server. They can be divided into independent systems. These independent systems are classified into data collection, content indexing, content analysis, content navigation, content search, user analysis, and content recommendation.
Project Information
- Content and preference data platform: Managing collection and storage of broadcast metadata and related information at KBS for content analysis, user profile information for broadcast analysis, broadcast service history, and user feedback information.
- Recommended Search Platform: By analyzing the content consumption history data of the user, the degree of similarity in patterns between two users who consumed different kinds of content is derived, and the subsequent content recommended search result is provided.
- Recommended search algorithm development: Two algorithms are implemented. One is a content-based algorithm that recommends content based on a user's input (search term, menu, content). This is done by analyzing the similarity or association between contents based on the characteristics of the collected content metadata. The other is a recommendation algorithm based on users' personal preferences and profiles. It uses a user-based collaborative filtering technique and an item-based collaborative filtering technique.
- Recommended Search Management: Implementing data and service management features required for recommendation systems, such as collection management, index management, project management, API management, and content management.
- Recommended Search Utilization Service: Develop recommendation search interface and implement utilization services such as program-related menu and related recommendation page, content/keyword/person-based recommendation search, customized recommendation work, and recent recommendation work.
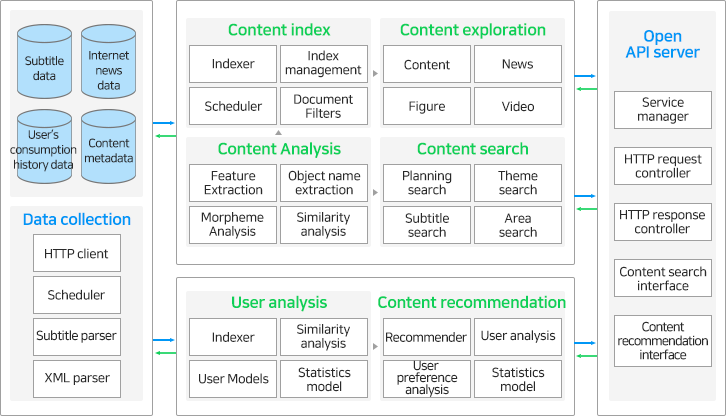
Application technology and solutions
Natural Language Understanding Engine and Complex Reasoning Engine.
Main accomplishments
- Identifying research trends of recommendation search for broadcasting content recommendation services. Based on existing recommendation system examples, plan, study, and develop components of recommendation systems, recommendation algorithms, target features, and services.
- Collecting broadcast content data, metadata, and user history information based on broadcast metadata standards modeling. User history information implements a recommendation model according to each recommendation algorithm, while broadcast content data and metadata implement a recommendation model using text mining and statistical techniques.
- Content recommendation search is applied to match the OHTV recommendation service. In addition, it is applied to recommendation search web pages to build utilization services that use the recommendation search system.
- The recommendation search engine is applied to KBS integrated CMS to study how to use the engine. We developed a user-based recommendation search service that provides five recommendation features: consumption history-based recommendation, preferred person recommendation, preferred genre recommendation, group/gender/age recommendation, and composite recommendation.
News and social content, which are the heart of big data, can be analyzed through artificial intelligence. Saltlux built Genie News service, a smart news app that provides and recommends personalized information to over 300,000 users by analyzing more than 1,200 online/offline news as well as social media in real-time using the AI engine.
Genie News AI engine reads 7 million news and blogs a day, just as people do. It automatically classifies them into more than 500 categories and recognizes emerging issues, thus providing customized service. In particular, the personalized news feature uses Deep Learning technology to learn what each user reads and registered keywords of interest, thus automatically predicting and recommending the content that users may like based on what the engine learned. It creates a kind of artificial user persona and personalized neural network in which content is recommended to each individual through an anonymous artificial neural network without collecting users' personal information.
Project Information
- Deep learning-based content recommendation customized for user: Study deep learning-based psychography technology and demography-based integration recommendation technology to develop news recommendation service.
- Intelligent curation of deep news and social content:Extracting core content and major issues(themes) through large-scale content real-time analysis.Classifying content and providing semantic technology-based related/similar content analysis.
- Providing more advanced user experience (UX): Implementing UI/UX that enhances user convenience and content readability.Personalizing interest content and implementing social sharing features
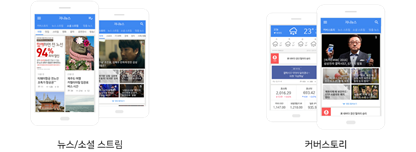
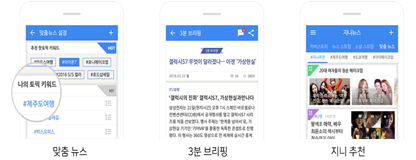
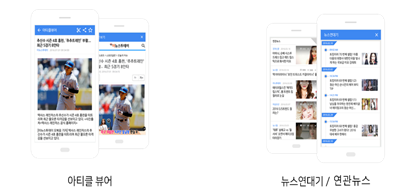
Application technology and solutions
Natural language understanding engine and complex reasoning engine
Main accomplishments
- (cover story) Providing real-time news for users and confirming today’s hot issues by providing card news, eliminating repeated news, and ranking news, just-ins, 3-min briefings, etc.
- (news/social stream) Collecting real-time news from major newspapers and providing various content, including social media content, by selecting categories by subject/topic.
- (Customized News) Based on the registered keywords of interest, select and provide content about relevant subjects that viewers are interested in, regardless of news or social media.
- (3-min briefing) Daily briefings with important information such as politics, economy, world, IT, and sports.
- (Ziny recommendation) analyzed preferences of individual users to provide contents that they may prefer. A variety of content by topics, ages, and genders, are provided using recommendation algorithms.
- (Article Viewer) Provides an ad-free smart view considering the user's convenience and readability.
- (News Chronicles/Related News) Articles are analyzed by date and automatically categorized in chronological order to be viewed at a glance. Providing a variety of news that the user might be interested in by recommending other related topic articles.
Saltlux introduced AI counseling systems and virtual counseling assistant services to the Japanese financial and aviation industries. We commercialized AI technologies such as machine learning, knowledge reasoning, and deep Q/A. The introduction of an artificial intelligence counseling system prevented customer churn, allowed the system to solve problems on its own, improved customer satisfaction, enhanced corporate image, increased operating profit, and increased customer satisfaction.
Project Information
- Mizuho Bank Virtual Counseling: We built a service with a concept of concierge in which, just like an actual counselor, the system understands the intentions of people's questions, finds appropriate answers, and provides those answers. Push-type navigator Mi-na service is provided on its business website to deal with Mizuho Bank customers' problems.
- Monex Securities's consultation services: Consultation services were added on the Monex Securities website.
- ‘Daily Guardian’ service at Nipponkoa Insurance Company’s official website: An ‘Daily Guardian’ service was set up at Nipponkoa Insurance Company’s official website to help deal with customers’ issues.
- Amy service on ANA SKY WEB of All Nippon Airways (ANA): Amy service was set up on ANA SKY WEB of All Nippon Airways (ANA, All Nippon Airways)
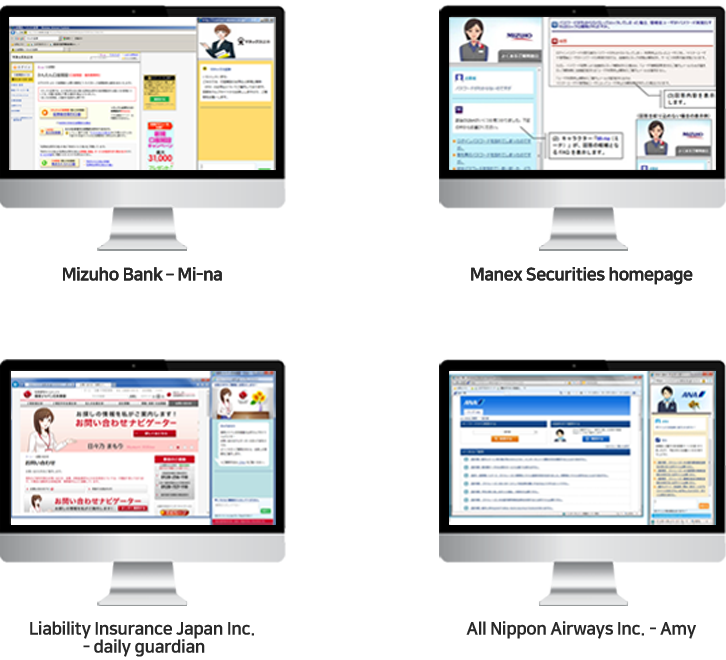
Application technology and solutions
Application of natural language understanding engine and deep Q/A engine.
Main accomplishments
- Prevent customer churn: Provide the customer with the necessary information to prevent the customer from leaving the service page.
- Decrease in the number of consultations: Reduce the number of consultation calls and inquiry emails by replacing call center content with Concierge guidance services.
- Improvement of the level of customer satisfaction: Improve quality by supplementing missing knowledge through service monitoring. Actual customer satisfaction increases due to the continuously rising rate of service users' reviews.